Robotic surgery
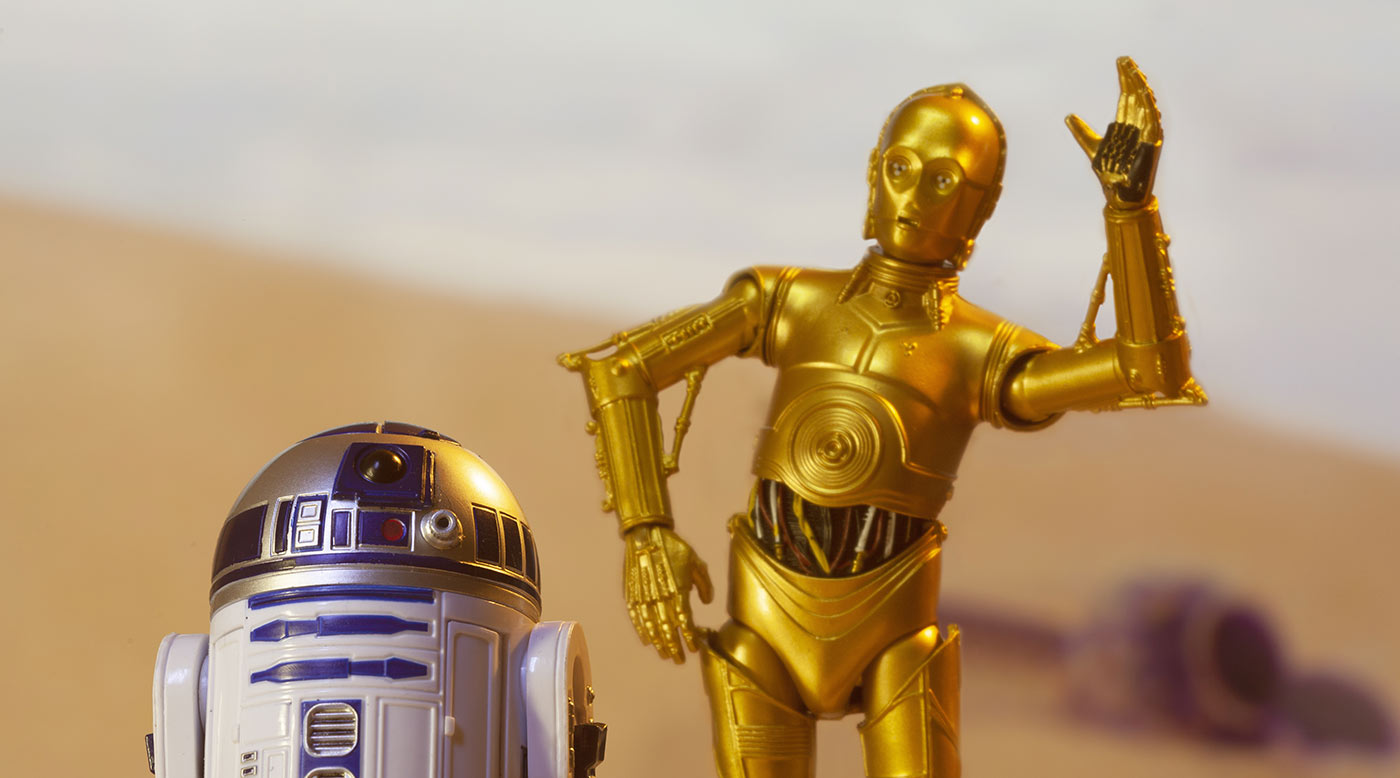
Written and edited by Nida Fatima, MD and Jakob Bower.
From SpaceX to Microsoft to the National Aeronautics and Space Administration (NASA), super intelligent machines and robots are considered the dominant form of intelligence on earth. However, robots were considered to be a matter of science fiction for approximately 100 years.
Robots were first described as dumb machines replacing monotonous work in the 1921 play Rossum’s Universal Robots (RUR) by Czech playwright Karel Capek. In 1950, Issac Asimov introduced us to an ultra-intelligent anthropomorphic robot in his classic science fiction book I, Robot.
Witty and sometimes deadly robots became blockbuster celebrities twenty-seven years later, as R2D2 and C3PO of Star Wars and Cyborgs of the Terminator film filled theaters worldwide.
Real life medical robots, on the other hand, were first envisioned by artist and technologist Scott Fisher in 19881. Fisher worked to integrate virtual reality with human interface technology and created a dexterous telemanipulator that greatly enhanced vascular and nerve anastomoses for hand surgery.
Since his study, robotics have revolutionized neurosurgical practice with their enhanced dexterity, automation, visualization, precision, and accuracy.1 Medical systems employing robots range from the passively remote-controlled to the fully automated, the latter using powerful imaging and computing technologies as a substrate to execute surgical plans under direct human supervision.
While there are major obstacles toward the broad adoption of robotic platforms in surgery, including the inherent complexity of surgical procedures and limited range of motion of robotic arms in interbody space, artificial intelligence (AI) is providing glimpses into a better future. AI-controlled algorithms combined with robotic devices can outperform humans in surgical tasks, reducing technical errors and surgical duration while accessing hard-to-reach body areas in transsphenoidal surgery2 and end-to-end bowl anastomosis3.
The development of autonomous robots by integrating computer vision, AI algorithms, and smart sensor technology is laying the foundation for a ‘modern surgery’ that will improve patient care worldwide. If we ensure the technology is reliable and accessible without discrimination, we may be able to achieve the highest standards of global health currently possible.
Medical robotics and virtual reality have the potential to train the next generations of surgeons, especially in developing countries where surgical training programs are scarce and standards are variable. Furthermore, these robots can provide excellent surgical care in areas with limited access to healthcare, such as war zones, disaster areas, spacecraft, and international space stations.
Smart sensors and AI algorithms will play critical roles in preventive medicine. Management plans for patients with chronic back pain and epilepsy can be generated using predictive probability algorithms trained on clinical and radiographic information. Continuous data collected from millions of people wearing medical sensors will be stored and analyzed by AI algorithms to inform us of new ways to understand and treat surgical disorders.
Circulating nano-sensors injected in spinal fluid will inform us of neurological pathologies long before they become radiologically or clinically evident. Post-operative monitoring and prevention of acute- and long-term complications will be made practically feasible by smart sensors.
The Autonomy Levels for Unmanned System (ALFUS) framework4, developed by the US National Institute of Standards and Technology, can form the operational foundation of an automated surgeon (Figure 1).
This autonomous robot will utilize sensing, decisional, and actuation capacities coupled with several algorithms to meet global standards of surgeon performance. A fixed set of sensors (e.g., odometrical encoders, range finder, stereo, laser, optical, haptic) are used to perform functions like mapping, localization, trajectory planning, complex tasks (e.g., suturing, knot tying, cauterizing, cutting), obstacle avoidance, and general supervision.
The robot’s operational domains and capacities are impacted by its environment, namely, the size and complexity of the task. This aspect in turn determines the energy consumption, execution time, reactivity, and robustness.
Actions taken by the autonomous surgical device are controlled by computer algorithms, directly programmed (explicit learning) or generated from observing human surgeons via machine learning (implicit learning), and are continuously reinforced and modified by domain experts5,6,7.
Following algorithm training, several simulations are performed to link the device’s performance to certain environmental complexities. In this way, we can compare robots with different functionalities and, depending upon the best performance, a robot with a unique algorithm suited for the task will be chosen.
This technology will perform surgeries beyond human limitations, making it a clinically effective tool. More importantly, it will be highly economical due to its prognostic efficacy, replicability, absence of human error, and proven clinical outcomes. Automated surgeons will provide fruitful and rewarding outcomes with the promise of greatly improving quality of patient care worldwide.
References:
- Kwoh YS, Hou J, Jonckheere EA, Hayati S. A robot with improved absolute positioning accuracy for CT guided stereotactic brain surgery. IEEE Transactions on Biomedical Engineering. 1988 Feb;35(2):153-60.
- Soldozy S, Young S, Yağmurlu K, Norat P, Sokolowski J, Park MS, Jane Jr JA, Syed HR. Transsphenoidal surgery using robotics to approach the sella turcica: Integrative use of artificial intelligence, realistic motion tracking and telesurgery. Clinical Neurology and Neurosurgery. 2020 Aug 11:106152.
- Shademan A, Decker RS, Opfermann JD, Leonard S, Krieger A, Kim PC. Supervised autonomous robotic soft tissue surgery. Science translational medicine. 2016 May 4;8(337):337ra64-.
- Huang HM, Messina E, Albus J. Toward a generic model for autonomy levels for unmanned systems (ALFUS). NATIONAL INST OF STANDARDS AND TECHNOLOGY GAITHERSBURG MD; 2003 Sep 1.
- Panesar S, Cagle Y, Chander D, Morey J, Fernandez-Miranda J, Kliot M. Artificial intelligence and the future of surgical robotics. Annals of surgery. 2019 Aug 1;270(2):223-6.
- Fatima N, Massaad E, Hadzipasic M, Shankar GM, Shin JH. Safety and accuracy of robot-assisted placement of pedicle screws compared to conventional free-hand technique: a systematic review and meta-analysis. Spine J. 2021 Feb; 21(2):181-192.
- Fatima N, Zheng H, Massaad E, Hadzipasic M, Shankar GM, Shin JH. Development and Validation of Machine Learning Algorithms for Predicting Adverse Events After Surgery for Lumbar Degenerative Spondylolisthesis. World Neurosurg. 2020 08; 140:627-641. PMID: 32344139.