How to start understanding Machine Learning in spine research
BY DR WONGTHAWAT LIAWRUNGRUEANG
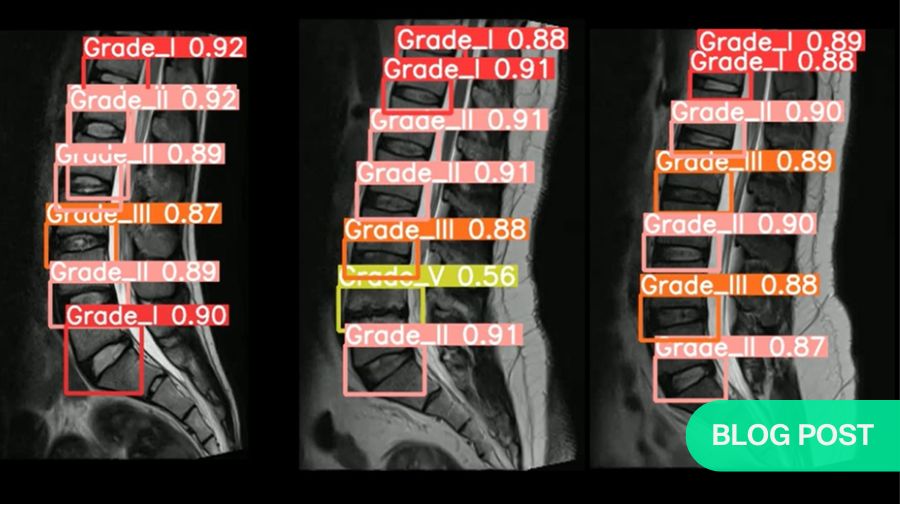
Machine learning (ML) is transforming spine research, particularly in areas like diagnosis and outcome prediction. One of the key applications of ML in spine surgery is enhancing the diagnosis of conditions like lumbar degenerative disc disease (DDD).
This blog post offers a beginner's guide to understanding machine learning and the possibilities it opens in spine research, with real-world examples from a review conducted on AI-assisted MRI diagnosis in lumbar DDD.1,2
Disclaimer: The article represents the opinion of individual authors exclusively and not necessarily the opinion of AO or its clinical divisions.
There are numerous hot topic discussions and dedicated conferences on artificial intelligence (AI) and machine learning, including a session on AI and ML at AO Spine's annual Global Spine Congress 2024 in Bangkok. Machine learning was a prominent topic at the GSC as it is a critical focus area in spine research. Understanding the fundaments will help to follow and participate in the ongoing discussion.
Why is Machine Learning important in spine research?
ML models can analyze MRI images to detect conditions like disc degeneration more accurately than traditional methods. For instance, current AI-assisted diagnostic applications have demonstrated that AI models can achieve up to 95% accuracy in detecting and grading lumbar DDD, highlighting the potential of ML in clinical settings.
Additionally, AI models automate tasks such as detecting lumbar disc herniation, bulging, and stenosis from MRI scans, saving clinicians valuable time and improving workflow efficiency.3
How to start the journey in Machine Learning for spine research?
Getting started with something new can feel daunting, but taking a few simple steps will help you get started in understanding and using ML in spine research:
1. Learn the basics: Start with online courses in AI and machine learning (Figure 3), focusing on key concepts like supervised learning and deep learning (e.g., convolutional neural networks, CNNs). These are commonly applied in spine research for tasks like detecting lumbar disc degeneration.
2. Understand relevant algorithms: Familiarize yourself with algorithms used in spine research, such as CNNs for image-based diagnosis (e.g., MRI), Random Forests for predicting patient outcomes, and Support Vector Machines (SVMs) for classifying healthy vs. degenerated discs.
3. Work with public datasets: Practice with spine MRI datasets from sources like Kaggle or the NIH. These datasets often come with annotations, allowing you to train and test ML models.
4. Data preprocessing: Learn how to clean and augment data to improve model performance. This involves normalizing MRI images and expanding datasets using data augmentation techniques.
5. Collaborate and experiment: Partner with data scientists or clinicians to annotate and analyze data and use tools like Labelbox for manual image labeling. Start small with simple models before progressing to more advanced applications.
Case study: AI in diagnosing Lumbar Degenerative Disc Disease
This review showcases how AI models, specifically CNNs, were used to diagnose and grade lumbar DDD. These models consistently outperformed traditional diagnostic methods, achieving accuracies as high as 99.5% in certain studies.
This exemplifies how ML can be applied in spine research to enhance diagnostic precision (Figure 4).1,3
Key concepts to understand
- Supervised learning in action: Supervised learning involves training a model with labeled data. In the case of lumbar DDD, AI models were trained using MRI images labeled with the Pfirrmann grading system to classify disc degeneration.4This approach resulted in high diagnostic accuracy across multiple studies.
- Deep learning and Convolutional Neural Networks (CNNs): Deep learning techniques, such as CNNs, were employed to automatically extract features from MRI images, enabling accurate identification and classification of lumbar disc pathologies. CNNs in this context proved effective in detecting subtle changes in disc morphology that may be missed by human interpretation.
- Performance metrics: The performance of ML models in lumbar DDD diagnosis was evaluated using metrics such as accuracy, sensitivity, and specificity. For example, the CNN model used by Liawrungreung et al. achieved an accuracy of approximately 95% in detecting lumbar intervertebral disc degeneration.
Finally, ML offers powerful tools for advancing spine research, particularly in diagnosing complex conditions like lumbar DDD. As demonstrated by AI-assisted models in recent studies, ML has the potential to improve diagnostic accuracy, streamline clinical workflows, and ultimately enhance patient care.
By starting with real-world applications and collaborating with AI experts, you can leverage the power of machine learning in your spine research.
About the author:
Dr Wongthawat Liawrungrueang is the Assistant Vice President (Foreign Affairs) of the Spine Society of Thailand (SST), Chair of the Spine Division, and Head of the Orthopaedics Department at the University of Phayao. Wongthawat conducts research in the fields of minimally invasive spine surgery, full-endoscopic spine surgery, artificial Intelligence, and software engineering.
He currently serves as a board member of the Asia Pacific Spine Society (APSS) and as faculty for AO Spine.
References:
-
Liawrungrueang W, Kim P, Kotheeranurak V, et al. Automatic Detection, Classification, and Grading of Lumbar Intervertebral Disc Degeneration Using an Artificial Neural Network Model. Diagnostics (Basel) 2023;13(4):663.
-
Liawrungrueang W, Cho ST, Sarasombath P, et al. Current Trends in Artificial Intelligence-Assisted Spine Surgery: A Systematic Review. Asian Spine J 2024;18(1):146–57.
-
Liawrungrueang W, Park J-B, Cholamjiak W, et al. Artificial Intelligence-Assisted MRI Diagnosis in Lumbar Degenerative Disc Disease: A Systematic Review. Global Spine J 2024;21925682241274372.
-
Pfirrmann CW, Metzdorf A, Zanetti M, et al. Magnetic resonance classification of lumbar intervertebral disc degeneration. Spine (Phila Pa 1976) 2001;26(17):1873–8.
You might also be interested in:
Global Spine Congress (GSC)
The GSC is one of the largest spine meetings globally, bringing together thousands of spine surgeons from around the world to share knowledge and discuss the latest hot topics.
Global Spine Journal Special Collections
The collections contain handpicked articles from various issues and provide an organized and efficient way to find and read articles by topics such as AI and Novel Technologies.
AO Spine members get more
Join the leading global academic community in spine care. Becoming an AO Spine member is just the beginning! Find out what's in store for you and how you can develop your career.
Artificial Intelligence and Machine Learning in spine surgery
A potential game changer! Sameh Abolfotouh delves into the hot topic revolutionizing countless industries; one of them may well be spine surgery.